.webp)
Published on
January 1, 2025
What is Fraud Detection? Importance, Best Practices & Challenges in 2025
In this story
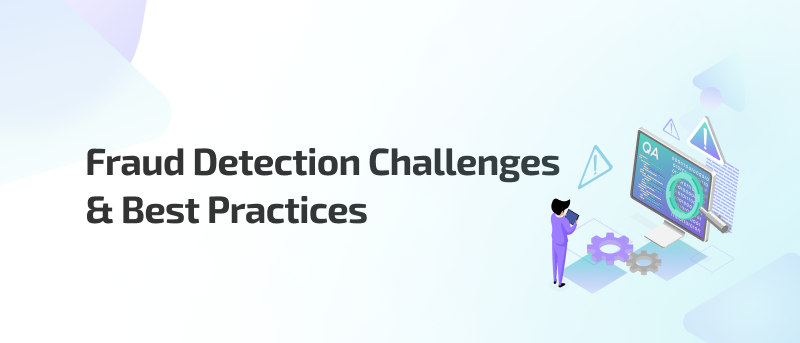
Accelerate AML Compliance: Meet Regulatory Demands with 80% Less Setup Time
Fraud poses one of the top threats facing digital finance today, causing massive financial losses plus reputational damages. According to estimates, global fraud rates can range from 1-3% of revenues - translating to billions in costs to the banking and fintech sectors. At the same time, evolving technologies like cloud, AI and digitization facilitate new forms of fraud that bypass traditional monitoring systems dependent on rules.
To stay secure as well as compliant, financial institutions need advanced fraud management capabilities. Powered by innovations in big data, machine learning, and collective intelligence, modern fraud systems can spot anomalies and suspicious activities that point to fraud at precursor stages before significant impact. Automated AI systems also allow scaling fraud monitoring to handle millions of transactions with improved detection precision levels over human efforts. This article will provide a comprehensive overview of modern fraud detection and prevention for digital finance - covering the scale of the challenge, leading techniques, best practices, and persisting pain points institutions face in combating fraud today.
Explore the secrets to protecting your business from fraud in our must-read Fraud Prevention E-Book
What is Fraud Detection?
Fraud detection refers to strategies and tools used to identify fraudulent activities, transactions, and threats across digital environments. It aims to prevent financial losses and data breaches by spotting illegal actions as early as possible.
Some examples include detecting:
- Fake accounts
- Identity theft
- Phishing scams
- Payment fraud
- Account takeovers
Effective fraud detection is essential for banks, ecommerce, fintechs, and any online business to limit fraud exposure and maintain trust & compliance.
Comply quickly with local/global regulations with 80% less setup time
Why Is Fraud Detection Important?
There are several key reasons why effective fraud detection is crucial for all digital businesses and financial service providers:
1. Prevent Massive Financial Losses
Fraud causes massive direct revenue losses for both banks and merchants - to the tune of billions annually. Estimates suggest average fraud rates of 1-3% of transactions for businesses. Digital payment fraud alone caused $42 billion in losses in 2021. Early detection through automated AI systems can stem fraud in the early stages before further abuse and losses.
2. Maintain Customer Trust
Rising undetected fraud undermines consumer trust and adoption of digital financial services and online commerce. Research reveals a majority of consumers are already concerned about digital fraud risks to their personal data and finances. Effective fraud prevention reassures users that their providers have invested in protecting their sensitive data plus assets.
3. Avoid Regulatory and Legal Penalties
With multiple state, federal, and international authorities now focused heavily on consumer protection in digital finance - insufficient fraud prevention can expose businesses to hefty regulatory fines for non-compliance plus substandard security.
4. Reduce Reputational Damage From Data Breaches
Fraud efforts like credential stuffing or phishing often serve as precursor steps to major data thefts. Detecting and blocking fraud intrusions early prevents attackers from gaining footholds within IT systems - reducing the likelihood of destructive data breaches down the line.
Industries Using Fraud Detection Systems
Many major industries now leverage AI-powered fraud detection systems and solutions to enable risk monitoring, including:
1. Banking
- Retail banking - Detect payment/transaction fraud, account takeovers, new account fraud, and loan fraud.
- Commercial banking - Prevent business account compromise, wire transfer fraud, commercial lending fraud
- Investment banking - Monitor insider trading activities, fraudulent reports/disclosures
2. Financial Services
- Insurance - Catch application fraud, claims fraud, multi-claim fraud, identity fraud
- Credit cards - Identify transaction fraud, bust out fraud, identity theft, loyalty program abuse
- Stock trading - Detect market manipulation attempts, insider trading
3. Digital Commerce & Payments
- Ecommerce - Spot e-commerce payment fraud, fake user accounts, promotions abuse, chargeback fraud
- Fintech - Prevent fraud across digital loans, wallets, crypto payments, wealth platforms
- Digital media - Block ad fraud, fake accounts, bot traffic, affiliate scheme fraud
4. Telecommunications
- Mobile/cellular networks - Detect SIM swap fraud, IRSF routing fraud
- Landline networks - Identify PBX hacking for call hijacking+IRSF fraud
5. Critical Infrastructure
- Energy/smart grids - Prevent automated bot attacks on IoT meters
- Water systems - Detect any tampering or backdoor access attempts
6. Government Agencies
- Taxation - Prevent fraudulent refund payouts, identity-related fraud
- Welfare - Spot multiple claims from single beneficiaries
- Border control - Detect identity fraud by travelers or immigrants
- With digital transformation touching so many sectors, the need for automated and robust fraud management capabilities continues to grow exponentially.
Regulatory Compliance
Fraud detection is also vital for regulatory compliance in the digital finance sector. Some key regulations driving the adoption of fraud monitoring:
- AML - Anti-money laundering protocols require tracing illegal transactions.
- PSD2 - Requires stepped-up identity checks and fraud screening.
- GDPR - Demands protections against data breaches perpetuated via fraud.
- PCI DSS - Calls for secured handling of payments and card data.
- SOX - Mandates financial control & transaction monitoring.
Advanced Techniques for Fraud Detection Systems
With digital fraud evolving quickly, fraud systems require robust technology to detect emerging and unknown fraud patterns accurately. Main techniques include:
- Artificial Intelligence - AI models using advanced ML algorithms can uncover complex frauds human investigators miss and adapt detection to new fraud schemes.
- Behavioral Analytics - Analyzing user behavior models like transactions, geolocations, and web/app patterns allows detection out of pattern anomalies pointing to fraud.
- Graph Analysis - Link analysis between entities reveals connections between accounts/devices to uncover fraud rings.
- Cloud Analytics - Cloud infrastructure enables analyzing millions of transactions with high speed and precision essential for real-time fraud prevention.
10 Best Practices for Fraud Detection
Implementing effective fraud detection calls for the following security best practices:
1. Use AI and Machine Learning Models: Rules-based systems have limited efficacy today. AI and ML models can detect novel fraud patterns and adapt dynamically. Continually retrain models on new data.
2. Enable Pre and Post-Transaction Monitoring: Spot fraud precursor signals during sessions and transactions while also analyzing post-activity forensics.
3. Adopt Risk-based Identity Authentication: Use step-up auth measures like 2FA selectively based on access risk to balance UX and security.
4. Leverage Graph Databases: Map relationships between entities to uncover linked fraud events and coordinated attacks.
5. Build Cross-Channel User Profiles: Create holistic profiles tying user behavior across web and mobile devices to detect sophisticated cross-channel fraud.
6. Create Fraud Data Sharing Consortiums: Collaborative inter-bank data exchange on threats improves detection for the whole industry.
7. Conduct Continuous Penetration Testing: Regular red team tests by specialized security firms can uncover detection gaps to address.
8. Develop Automated Case Management: Use AI for fraud analysis and to aid investigators with intelligence alerts and document retrieval.
9. Adopt agile Model Development: Short, iterative cycles allow moving fast to tackle new fraud patterns through rapid prototyping.
10. Leverage External Data: Enrich in-house signals with AI-processed alternative data like identities, devices, locations, and deep web. The key is combining adaptive AI, collective data, and continuous system improvement to create a fraud infrastructure capable of evolving at pace with the fraud threats.
The 5 Main Challenges of Fraud Detection
While critical, fraud prevention faces persisting technology and organizational barriers:
- Detection at scale: Daily transaction volumes in banking and fintech can overwhelm conventional fraud systems. Requires rethinking data architectures.
- Increasing fraud sophistication: Evolving attacks like social engineering, botnets, and malware circumvent traditional rule-based detection dependent on known fraud signatures.
- Limited cross-channel visibility: Most institutions have channel siloed detection, unable to correlate user behavior across web, mobile and payments for better signals.
- Difficulty building holistic user profiles: Restricted sharing of security events across banks as well as internal silos creates blind spots in user activity modeling.
- Accessing unambiguous fraud ground truth: Deep neural networks need large training data sets with confirmed fraud cases - but these events are often not documented clearly by fraud teams.
How to Detect Fraud with FOCAL AI
FOCAL AI provides a next-gen cloud platform for enabling end-to-end fraud detection and response across banking, fintech, and digital finance sectors.
Capabilities include:
- Omni-Channel Detection: Correlate user behavior across web, mobile, and payment channels to accurately detect sophisticated frauds spanning channels.
- Powerful AI Engines: Deep learning algorithms continuously model evolving fraud tactics to maintain high precision rates.
- Case Management: Tools for fraud teams to efficiently investigate threats and collaborate on incident response.
- No Code Setup: Pre-built industry templates allow quick setup without complex coding or data science skills.
- IP Threat Intelligence: Block common fraud IPs or connections leveraging global fraud databases. Integrated with a 3rd party IP database that's updated continuously with the most up-to-date list of IPs.
- Built-in Best Practices: Embedded expertise from detecting financial fraud worldwide.
FOCAL AI also offers fraud monitoring use cases covering payment fraud, account takeover protection, synthetic identity detection, and application fraud for banking and fintech sectors.
Conclusion
As digital finance grows globally, fraud management is crucial for minimizing revenue losses and maintaining consumer trust. AI and cloud-based detection enable modern systems to tackle both the volume and sophistication of emerging financial fraud. Best practices backed by advanced analytics and collective intelligence allow institutions to improve fraud resilience. Platforms like FOCAL AI deliver quick time-to-value by making state-of-the-art detection accessible without a complex setup.
FAQs
Q1: What Are Some Common Examples Of Fraud?
Some of the most common fraud types include payment fraud, account takeover fraud, new account fraud, identity fraud, insurance claims fraud, transaction laundering fraud, and more.
Q2: How Can Fraud Detection Lower Costs?
Early and automated fraud detection minimizes losses by preventing future abuse of compromised credentials or accounts. It also reduces the costs of manual investigations through AI automation. Preventing fraud incidents further avoids costs from data breaches and regulatory fines.
Q3: What Fraud Detection Capabilities Do Banks Need?
Banks need capabilities to monitor payment fraud, account takeovers, new account fraud, transaction anomalies, mule activity, wire transfer risks, employee fraud threats, bust-out fraud, loan fraud, identity spoofing, and more across digital and core banking channels.
Q4: Does Fraud Detection Improve Customer Experience?
Yes, good fraud detection improves CX. Frictionless risk-based authentication stops genuine customers from dropping off while stepping up security for high-risk events provides cover from fraud damages. Detecting and blocking fraudsters also gives customers confidence in the platform's security.
Q5: What Data Is Required For Fraud Detection?
Transaction history, authentication patterns, device prints, geolocation history, web session records, entity relationships, and other cyber-surveillance data need correlation to uncover fraud signals. External data also provides additional monitoring context.
Streamline Compliance: Achieve 80% Faster Setup for Fraud Prevention

How Aseel reduced onboarding time by more than 87% using FOCAL
Learn how FOCAL empowered Aseel to achieve new milestones.
Mastering Fraud Prevention: A Comprehensive Guide for KSA and MENA Businesses
51% of organizations fell victim to fraud in the last two years, don't be caught off guard, act proactively.
Featured blog posts
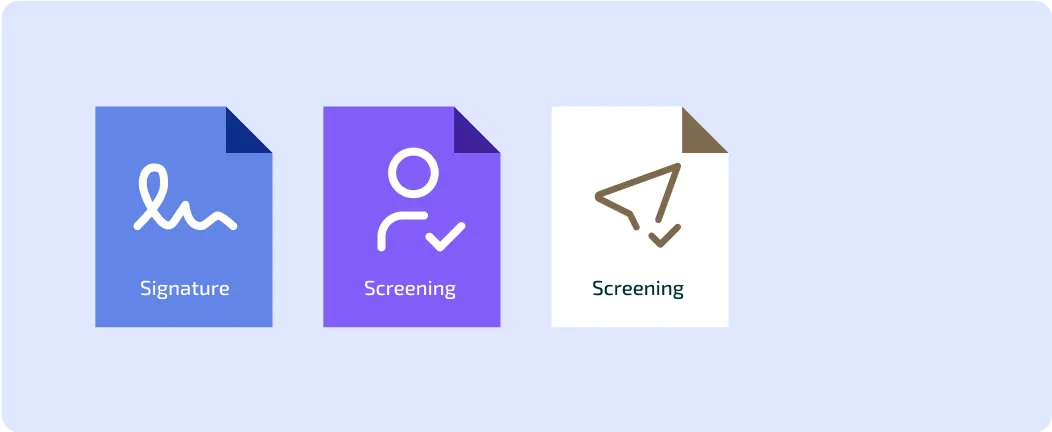
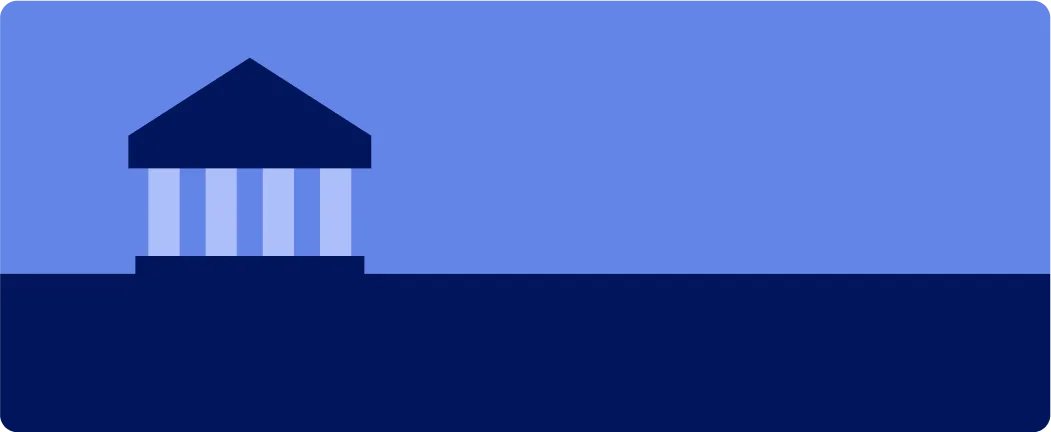

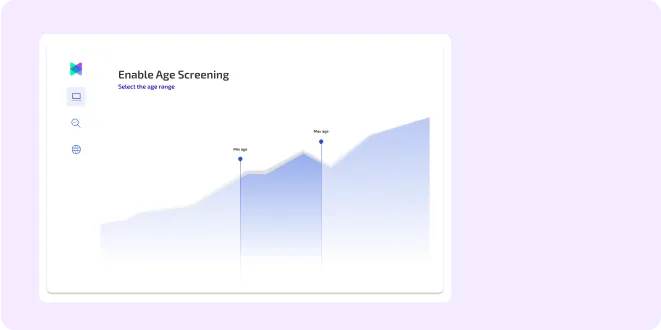
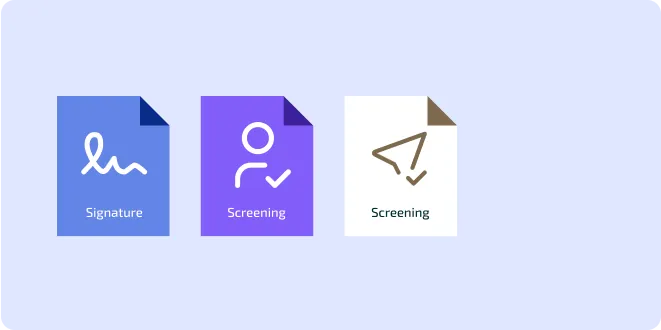
Comments
Leave a Reply
Comment policy: We love comments and appreciate the time that readers spend to share ideas and give feedback. However, all comments are manually moderated and those deemed to be spam or solely promotional will be deleted.