.webp)
Published onÂ
April 3, 2024
What is Customer Analytics? Types, Significance, Metrics in 2024
In this story

Accelerate AML Compliance: Meet Regulatory Demands with 80% Less Setup Time
Why do some businesses earn more money than others? Can using customer data really help businesses grow? According to McKinsey, businesses using customer data are three times more likely to increase revenue and twice as likely to get better results from their marketing investments compared to their rivals.
If you want a guide that explains all about customer data analysis, you've found it. By the end of this guide, you'll know how to use customer information and data analysis to do better than your competitors.
What is Customer Analytics?
Customer analytics, also called customer data analysis, means looking at a company's customer information and behavior to identify, attract, and retain profitable customers.
This includes gathering and understanding data from different sources & touchpoints like websites, applications, social media, and feedback surveys. Then, the data is studied to figure out what customers want, like, and expect. The outcome of this analysis helps businesses improve & optimize ads, understand the customer journey, and make personalized marketing plans.
Customer Analytics Metrics & Indicators
Key numbers and signs are really important in understanding customers and their behavior & interactions with a business. Here are four main customer analytics metrics:
- Customer Lifetime Value (CLV): CLV shows how much money a business can expect from one customer during their entire time with the company. It looks at how often they buy, how much they spend each time, and how long they stay. CLV helps businesses know how important it is to get and keep customers, which helps them decide how to market to customers and keep them coming back.
- Customer Acquisition Cost (CAC): CAC is how much a business spends to get a new customer. This includes but not limited to marketing, advertising, and sales efforts. By comparing CAC to CLV, businesses can see how well they are at getting new customers and if it's worth the money they spend, similar to the concept of return on investment.
- Churn Rate: The churn rate shows how many customers stop using a company's products or services in a certain time. High churn might mean customers aren't happy or there are problems or issues with the products and or services. When you track the churn rate, you can find out why customers leave and make plans to keep & retain them.
- Customer Segmentation: This means categorizing customers into groups based on things they have in common, like age or what they buy. It helps you make marketing and products that fit each group better.
Read more: Fraud Analytics: Identifying and Reducing Fraud Risks in 2024
How Does Customer Analytics Work?
The importance of consumer analytics lies in its ability to provide actionable insights that drive business growth and customer satisfaction. Consumer analytics data comes from different sources & touchpoints. The process occurs in order to understand how customers behave, what they like & dislike, as well as the trends they follow. Customer analytics happens in six steps:
1. First, the tool gathers data from various touchpoints like sales records, website clicks, social media, customer feedback, and more. This information comes from sources like CRM systems or market surveys.
2. After the data gets collected, there must be some sort of space for it to be stored and put together, to get the full picture of each customer.Â
3. Now, not all data is as useful and or accurate, hence the information & data collected needs fixing and to be "cleaned" before the analysis stage. During the data cleaning and preparation stage, the raw data which might have incorrect information gets corrected to be accurate and reliable and organized.
4. The forth stage is all about analyzing the data, this could mean summarizing through the descriptive analysis method, or using predictive analytics to anticipate and predict what might happens next, or suggesting the best plans based on those expectations which is the prescriptive analysis technique.
5. At the fifth stage, you start learning from this data, you could find categorizations and groups of customers that have similar behavior, you could get insights about your customers' preferences, and you can also predict future trends and inform business decisions and strategies. The benefits of these insights are enormous if the data is studied carefully.
6. The last and sixth stage comes the continuous improvement. Your methods and strategies should not get still and stone, on the contrary, you'll notice that there always will be areas for improvement and refinement.
Comply quickly with local/global regulations with 80% less setup time
Data Collection Methods
As we mentioned before, data is collected from various sources, and these sources can be internal or external:
- Data from the inside: This type of data is generated and kept in the company, and it includes but is not limited to sales records and website behavior.
- Data from the outside: This data comes from any source but the company. It might be reports on what people buy, information from social media, facts and demographic data, third-party data providers, and or economic indicators.
It is important to note here that no source is better or is more preferable than the other, the inside and outside data both complement each other to draw the full picture, they both provide additional context and insights into market trends, what competitors are doing and how people behave.
Data Processing and Customer Analytics Techniques
It is important to answer the question of ‘how to analyze customer data?’ In simple terms, customer data analytics looks back at data, uses this data to predict the future, and then also uses the data and outcomes to improve. Each of these types of customer analytics has a different analytic technique:
- Descriptive analytics looks at past data to see what happened before. It summarizes and shows data trends, patterns, and how things are connected. This helps understand how customers act and gives a base for more complex analytics.
- Predictive analytics uses math and computer learning to guess what might happen next based on past info. It looks at patterns in the data to predict future things like what customers might buy, if they might stop using a service, or what they might be interested in. Customer analytics models like clustering and predictive models help businesses forecast customer behavior and trends.
- Prescriptive analytics goes beyond predicting and gives ideas on what to do. It uses algorithms to suggest the best moves to get the outcomes a company wants. This helps make smart choices, like how to keep customers happy, how to make ads work better, or how to make the business run smoother.
Tools and Technologies for Customer Analytics
Customer analytics software helps businesses analyze customer data to understand behaviors and preferences effectively. Gathering data is not an easy thing to do, especially if you're operating on a large scale with a large number of clients and/or you have various touchpoints from which to collect the data. Hence, the technological tools and platforms that do so fast, and continuously.
1. Customer Relationship Management (CRM) Systems
A CRM stores various important details for your business, bank, financial institution, you name it. These details include names, contact information, purchase history, preferences, and a lot more!
It also tracks interactions between your organization and the customers or potential ones like phone calls, emails, and website visits. This information can boost customer relationships and sales and marketing efforts for your company or business. Customer analytics applications are crucial for improving marketing strategies and enhancing customer experience across industries.
Business customer analytics helps enterprises gain actionable insights into B2B customer behaviors and preferences. Customer analytics KPIs measure metrics like customer acquisition cost and customer retention rate to gauge business performance.
2. Business Intelligence (BI) Tools
Business analytics tools are very helpful for banks and other financial institutions. The bank actually can identify patters such as which products are the most popular amongst different customer categories and how customers respond to marketing campaigns. They also help the bank to know when certain financial services are in high demand.Â
3. Data Visualization Platforms
Why would the bank or a financial institution want to turn data into pictures and graphs? Well, visual representation of data makes it simpler for people to understand the complex data and hence make informed decisions based on it. So the bank utilized these data visualization platforms to create interactive dashboards, and in these dashboards, you can see metrics like customer acquisition rates, loan approval times, branch performance, & a lot more! A bank manager, for example, can easily view and assess performance trends, and identify areas for improvement.Â
How To Leverage Customer Analytics for Business Growth?
Customer data analytics is pivotal in driving business growth by providing insights that enable businesses to enhance their marketing strategies, product development efforts, and customer retention initiatives. Here's how customer data analytics can be leveraged for business growth:
Personalized Marketing and Customer Experience
1. Targeted Advertising Campaigns: Businesses use customer data analysis to divide their audience into groups based on demographics, behavior, and preferences. This segmentation enables the creation of focused advertising campaigns tailored to specific customer groups, improving the efficiency of marketing strategies and maximizing returns on investment (ROI).
2. Tailored Product Recommendations: consumer analytics enables businesses to understand individual customer preferences and purchasing patterns. This information can be used to deliver personalized product recommendations to customers, improving cross-selling and upselling opportunities and enhancing the overall customer experience.
3. Customized Communication Channels: Through consumer analytics, businesses can identify the communication channels preferred by their customers, whether it be email, social media, or SMS. Companies can increase engagement and build stronger customer relationships by delivering messages through preferred channels.
Improving Product Development and Innovation
1. Spotting Market Trends and Opportunities: Customer data enables banks to better understand what not only their existing customers but also potential ones like and what's currently trending in the market. If you see where customers need more, and find new ways to create better services and products. This helps them make things that customers want. Maybe your bank's customers are interested in digital banking services, so this should tell you something, it should prompt the bank to develop a new mobile banking app, for example.Â
2. Using Feedback to Improve Products: If your bank or financial institution has an, let's say, Instagram account, every comment is feedback your bank should pay attention to. However, it can be a lot easier, faster, and more accurate to analyze feedback from surveys, reviews, and social comments using a data analytics tool. The tool enables you and your team to pinpoint areas for improvement. So if many customers are complaining about the complexity of the online banking interface, your priority should be simplifying the interface to be user-friendly based on this feedback.
Enhancing Customer Retention and Loyalty
1. Anticipating and Preventing Churn: Using consumer data analytics, businesses can detect signs of potential customer churn, like reduced activity or lower satisfaction ratings. By using predictive customer data insights, companies can take proactive steps to stop churn by solving problems, giving rewards, or creating personalized retention plans.
2. Rewards and Incentives for Loyalty: With customer analytics, businesses can recognize valuable customers and learn about their likes and actions. This data helps in creating loyalty programs and rewards customized for different customer groups, encouraging loyalty and building lasting connections.
3. Continuous Improvement through Feedback Loop: Customer analytics enables businesses to continuously monitor and analyze customer feedback to identify areas for improvement. By implementing a feedback loop, businesses can gather customer insights, make necessary adjustments to products or services, and measure the impact of these changes on customer satisfaction and loyalty.
Trends in Customer Analytics
Customer analytics continues to evolve, driven by technological advancements and changing consumer behaviors. Here are key trends shaping the field:
Artificial Intelligence and Machine Learning (AI/ML) Advancements
- Enhanced Data Processing: AI and ML technologies like the FOCAL platform enable more efficient and accurate analysis of large datasets.
- Predictive Analytics: Predictive models powered by AI/ML forecast future trends and behaviors, informing strategic decisions.
- Automation: AI-driven automation streamlines data processing tasks, freeing up resources for strategic initiatives.
Predictive Personalization and Hyper-Targeting
- Personalized Experiences: Predictive analytics drives tailored product recommendations and marketing messages, enhancing customer engagement.
- Hyper-Targeting: Businesses use predictive indicators to target specific customer segments with relevant marketing campaigns, maximizing ROI.
Ethical and Responsible Data Use
- Privacy and Security: Businesses prioritize compliance with data regulations and implement robust security measures.
- Transparency and Accountability: Transparent communication about data practices builds customer trust and demonstrates ethical conduct.
- Customer-Centricity: Businesses focus on providing value to customers through data-driven personalization while respecting their privacy and preferences.
How Can FOCAL Help?
The FOCAL platform provides advanced customer analytics for banks and financial institutions in various ways. First, its smart & strategic financial forecasting tools use advanced algorithms and predictive models to assess risk and forecast finances. For example, a bank could predict which customers might default on loans and adjust their lending policies accordingly.
FOCAL trend analysis capabilities can spot important patterns in customer activities so that the bank adjusts the strategies based on evolving market dynamics and customer behaviors. For example, if more customers start using online banking, the bank can invest more in digital services.Â
FOCAL also analyses current income, market trends, salary raises, and past performance, and then based on that FOCAL can predict a customer’s future earnings, so that your bank tailors products to fit customer needs, such as offering investment products to customers with rising incomes.
Not only that but also FOCAL customer analytics technologies streamline transaction analysis, making it easier for banks to handle large amounts of data quickly and get real-time insights.
When FOCAL helps you learn about a customer’s spending habits, your bank can suggest a suitable credit card, or might offer a credit card with travel rewards to a frequent traveler.
Lastly and most importantly, FOCAL is built to comply with industry standards and regulations to stay compliant and avoid legal issues and AML fines.
Final Thought
Recent customer analytics trends show a shift towards predictive modeling and AI-driven insights for better decision-making. Accurate customer analytics are essential for making precise business decisions and effectively targeting marketing efforts. To ensure the ethical and responsible use of customer data in analytics, businesses should prioritize data privacy and security, comply with relevant regulations such as GDPR and CCPA, obtain explicit consent for data collection and processing, be transparent about data practices, and use data in ways that provide value to customers while respecting their privacy and preferences.
Additionally, businesses should implement measures to prevent customer data insights misuse or abuse and regularly review and update their data practices to align with evolving ethical standards and regulations.
FAQs
Q1. What are some typical applications of customer analytics?
Some common customer analytics applications include segmentation analysis, churn analysis, lifetime value analysis, product recommendation engines, predictive analytics, and sentiment analysis.Â
Q2. How does customer analytics differ from traditional market research?
Customer analytics is distinct from traditional market research. While traditional methods gather data through surveys, focus groups, and interviews to understand market trends and consumer attitudes, customer analytics analyzes data from multiple sources like transaction records, website interactions, and social media. This approach provides insights into customer behavior and preferences.
Q3. What types of data are typically used in customer analytics?
Customer analytics utilizes various data types, including demographic information, transactional data, website interactions, social media engagement, customer feedback, and more. This data is collected from internal sources, such as CRM systems and sales records, and external sources, such as market research reports and third-party data providers.
Streamline Compliance: Achieve 80% Faster Setup for Fraud Prevention

How Aseel reduced onboarding time by more than 87% using FOCAL
Learn how FOCAL empowered Aseel to achieve new milestones.
Mastering Fraud Prevention: A Comprehensive Guide for KSA and MENA Businesses
51% of organizations fell victim to fraud in the last two years, don't be caught off guard, act proactively.
Featured blog posts
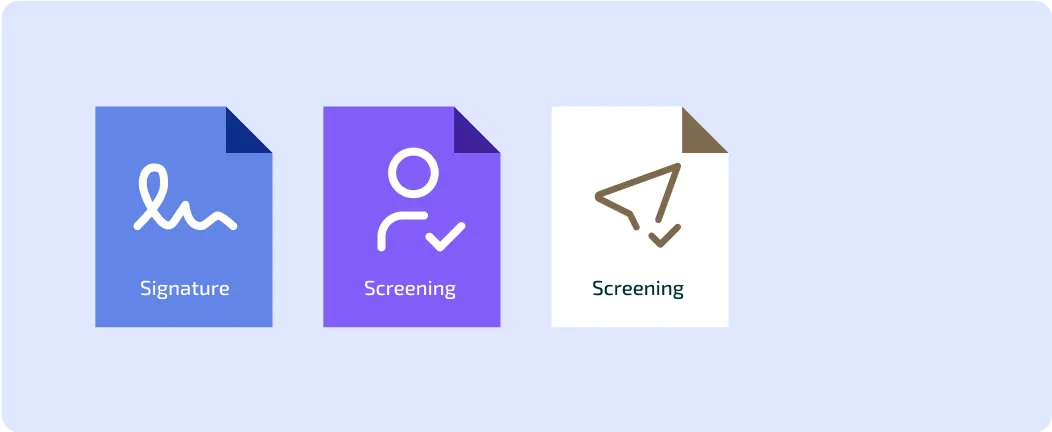
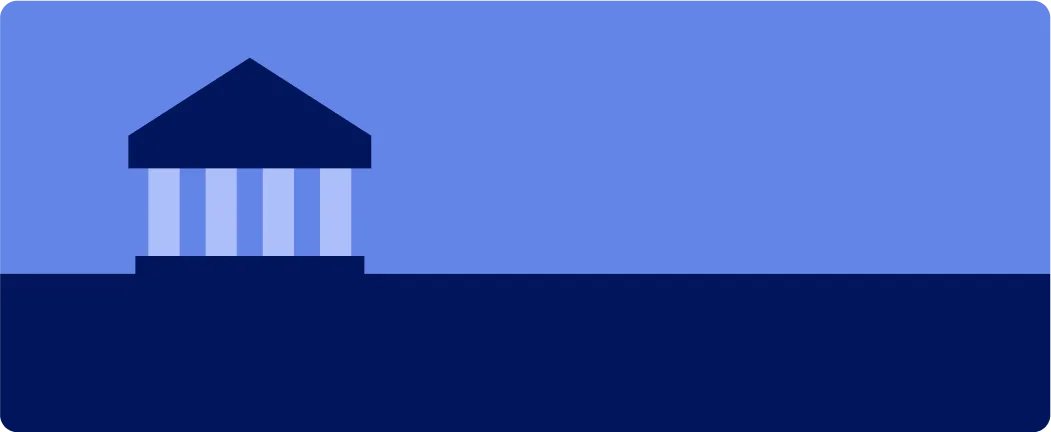

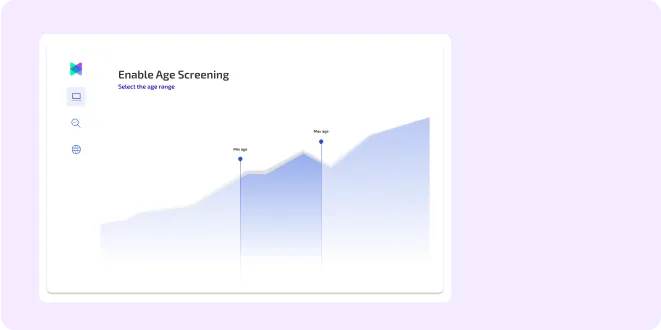
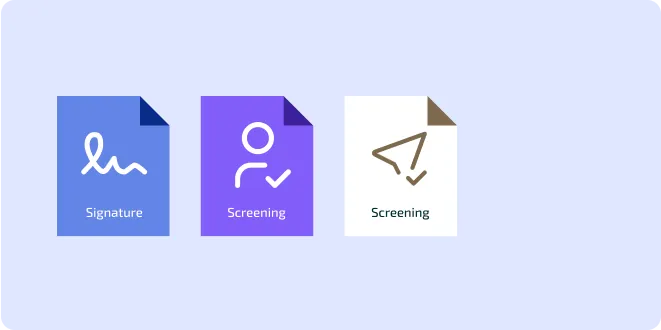
Comments
Leave a Reply
Comment policy: We love comments and appreciate the time that readers spend to share ideas and give feedback. However, all comments are manually moderated and those deemed to be spam or solely promotional will be deleted.