.webp)
Published on
July 28, 2024
Predictive Analytics in Banking: Importance and Use Cases
In this story

Accelerate AML Compliance: Meet Regulatory Demands with 80% Less Setup Time
The banking industry's global predictive analytics market is forecasted to grow substantially, with an estimated CAGR of 19.42% by 2030. This upward trend is propelled by the widespread adoption of the Internet of Things (IoT) technology, resulting in the proliferation of IoT-based systems worldwide.
An increase in illicit activities, including accounting fraud and money laundering, has also contributed to the expansion of the market. Predictive analytics in banking institutions have proven instrumental in predicting property payments and client flow, amongst others.
What is Data Analytics in Banking?
Data analytics plays a pivotal role in the banking sector, involving the examination of extensive datasets by financial institutions to extract valuable insights, detect patterns, and identify trends. This analytical process informs decision-making and aids in the optimization of various banking operations.
Also, data analytics enables banks to proactively assess and reduce risks, identify and prevent fraudulent activities, gain insights into customer behavior for personalized services, refine marketing strategies, and streamline operations for cost-effectiveness and productivity.
Key Components of Predictive Analytics
Banking predictive analytics requires understanding the key elements that form its foundation. Here is how you can build reliable insights from banking predictive analytics:
1. Gathering and Preparing Data
It starts with collecting relevant data from different sources and ensuring it's well-organized and accurate. Having reliable data is crucial for making reliable predictions.
2. Analyzing and Modeling Data
This step involves using statistical techniques and machine learning algorithms to analyze historical data and build predictive models. These models help identify patterns and trends that can be used to forecast future events or behaviors.
3. Selecting and Creating Features
Identifying important features or variables in the data and creating new ones can improve the performance of predictive models. The goal is to focus on the most relevant factors while minimizing noise.
4. Training and Evaluating Models
Predictive models are trained using historical data and evaluated to ensure accuracy and reliability. This may involve refining the models to improve their performance.
5. Implementing and Integrating Models
Once developed, predictive models are implemented into operational systems or integrated into decision-making processes. This allows for real-time predictions or decision support.
6. Monitoring and Maintaining Models
Continuous monitoring of model performance and data quality ensures that predictions remain relevant and accurate over time. Models may need to be updated or retrained periodically.
7. Interpreting and Applying Insights
Understanding the predictions generated by the models and translating them into actionable insights is essential. This involves incorporating predictive insights into business strategies or operations to drive value.
Read more: Fraud Analytics: Identifying and Reducing Fraud Risks in 2024
Comply quickly with local/global regulations with 80% less setup time
5 Types of Banking Analytics
Predictive analytics in retail banking is not the only type; analytics in retail banking has diverse forms of analytics, each serving distinct functions:
1. Descriptive Analytics: This type aims to summarize historical data, offering insights into past trends and performance within banking. Descriptive analytics forms the basis for more advanced analyses.
2. Diagnostic Analytics: This type delves deeper into historical data and uncovers underlying reasons behind past events or trends. It enables banks to understand the drivers of specific outcomes and improve performance.
3. Predictive Analytics: Focused on forecasting future outcomes or trends using historical data and statistical algorithms, predictive analytics is instrumental in anticipating customer behavior, assessing credit risk, detecting fraud, and optimizing marketing strategies in banking.
4. Prescriptive Analytics: Unlike predictive analytics, prescriptive analytics not only predicts future outcomes but also recommends actions to achieve desired objectives. In banking, it aids in optimizing decision-making by providing actionable insights based on predictive models and business constraints.
5. Cognitive Analytics: Advanced technologies such as artificial intelligence and natural language processing perform cognitive analytics, which helps analyze unstructured data sources such as customer feedback and social media. In the banking industry, cognitive analytics improves decision-making by providing valuable insights into customer sentiments, market trends, and emerging risks.
The Importance of Predictive Analytics in Banking Operations
Predictive analytics has transformative power in banking, and its significance spans different areas.
1. Risk Management
Banks face a variety of risks, including credit, market, and operational risks. Predictive analytics enables them to anticipate and address these risks by forecasting potential outcomes and spotting early warning signs of risk events.
2, Fraud Detection and Prevention
Fraudulent activities pose significant threats to both banks and their customers. Predictive analytics helps banks identify patterns of fraudulent behavior and detect suspicious transactions in real time, thereby preventing financial losses and protecting customers' assets.
3. Customer Insights
Understanding customer behavior, preferences, and needs is vital for banks to offer personalized services and enhance customer satisfaction. Predictive analytics allows banks to segment customers based on their attributes and predict future behaviors, enabling targeted marketing efforts and customized product offerings.
4. Marketing and Sales Optimization
Predictive analytics assists banks in optimizing their marketing and sales strategies by identifying the most effective channels, messages, and offers for different customer segments. This enables banks to attract and retain customers more effectively while maximizing profitability.
5. Operational Efficiency
Predictive analytics can improve operational efficiency by identifying inefficiencies, automating processes, and optimizing resource allocation. By streamlining operations, banks can reduce costs, minimize errors, and enhance overall productivity.
10 Use Cases of Predictive Analytics in Banking
Predictive analytics offers many practical applications within the banking sector:
1. Credit Evaluation: Banks utilize predictive analytics to assess the creditworthiness of potential borrowers by scrutinizing historical data to predict the likelihood of default.
2. Fraud Detection: Predictive analytics aids in real-time fraud detection by analyzing transaction patterns and identifying anomalies indicative of fraudulent activity.
3. Customer Segmentation: Banks categorize customers based on their attributes and behaviors, facilitating personalized marketing campaigns and product offerings.
4. Churn Prediction: Predictive analytics identifies customers at risk of leaving the bank by analyzing their historical data and behaviors. This, in turn, helps banks implement targeted retention strategies.
5. Cross-selling and Upselling: Banks harness predictive analytics to pinpoint opportunities for cross-selling and upselling by analyzing customers' past purchases and preferences.
6. Risk Management: Predictive analytics assists banks in managing various risks, such as credit, market, and operational risks, by projecting potential outcomes and detecting early warning signs of risk events.
7. Cash Flow Forecasting: Predictive analytics enables banks to forecast cash flows based on historical data and market trends to facilitate improved liquidity management.
8. Asset Liability Management: Predictive analytics aids banks in optimizing asset and liability management to predict shifts in interest rates and analyze customer behavior.
9. Market Analysis: Banks utilize predictive analytics to analyze market trends and anticipate changes in interest rates, exchange rates, and other economic indicators to inform investment decisions.
10. Customer Lifetime Value Prediction: Predictive analytics empowers banks to forecast the lifetime value of individual customers to enable targeted marketing efforts and customer retention strategies.
Conclusion
In conclusion, predictive analytics is a key asset in the banking industry, offering crucial insights and tools to boost operational efficiency, manage risks effectively, and enhance customer satisfaction. Through its application, banks can anticipate customer needs, identify fraudulent activities, optimize marketing strategies, and streamline internal processes.
Looking ahead, the future of predictive analytics in banking appears promising, with ongoing advancements in technologies like artificial intelligence and machine learning expected to further refine predictive modeling accuracy and capabilities.
Streamline Compliance: Achieve 80% Faster Setup for Fraud Prevention

How Aseel reduced onboarding time by more than 87% using FOCAL
Learn how FOCAL empowered Aseel to achieve new milestones.
Mastering Fraud Prevention: A Comprehensive Guide for KSA and MENA Businesses
51% of organizations fell victim to fraud in the last two years, don't be caught off guard, act proactively.
Featured blog posts
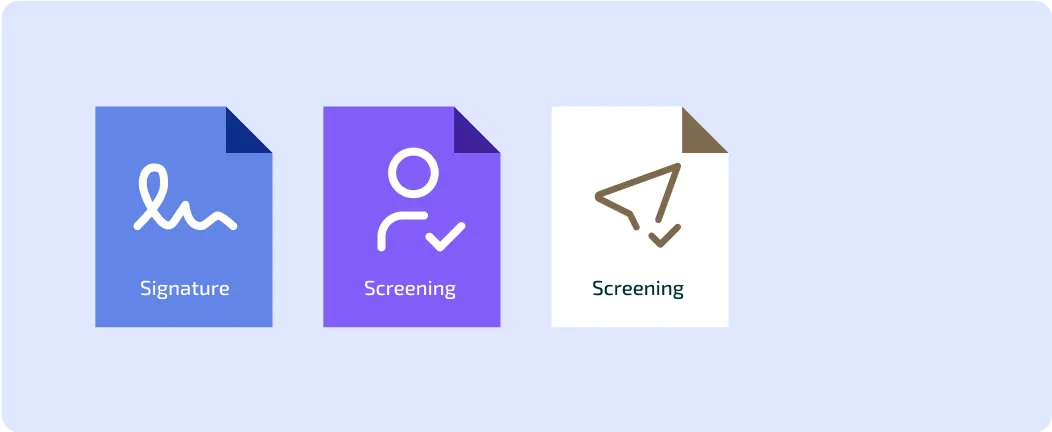
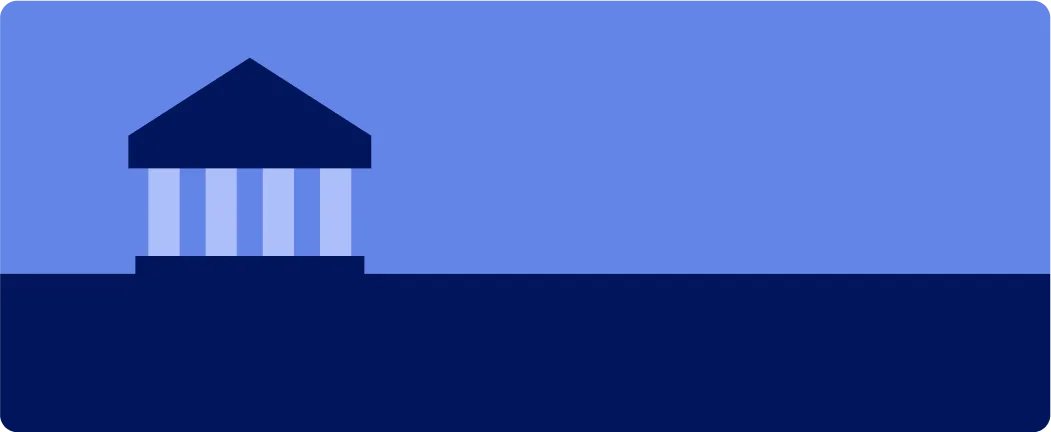

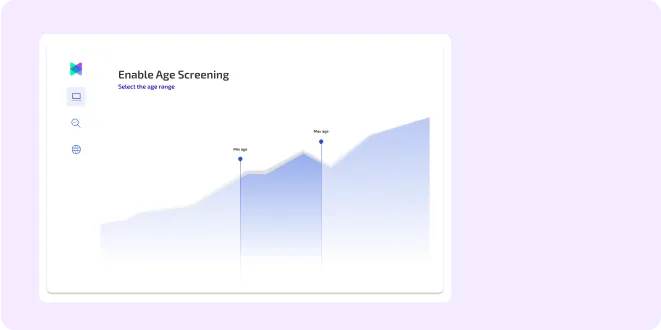
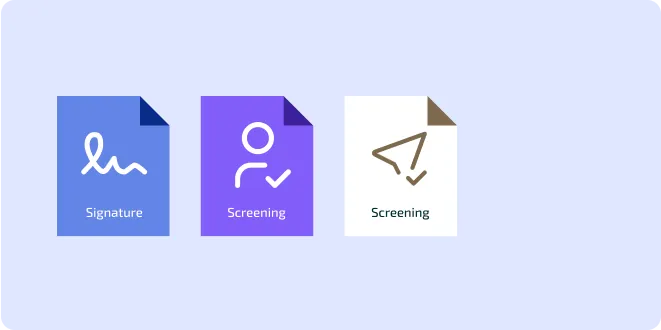
Comments
Leave a Reply
Comment policy: We love comments and appreciate the time that readers spend to share ideas and give feedback. However, all comments are manually moderated and those deemed to be spam or solely promotional will be deleted.