.webp)
Published onÂ
May 30, 2024
Mobile Fraud Detection: Identifying Types, Techniques, and Best Practices
In this story
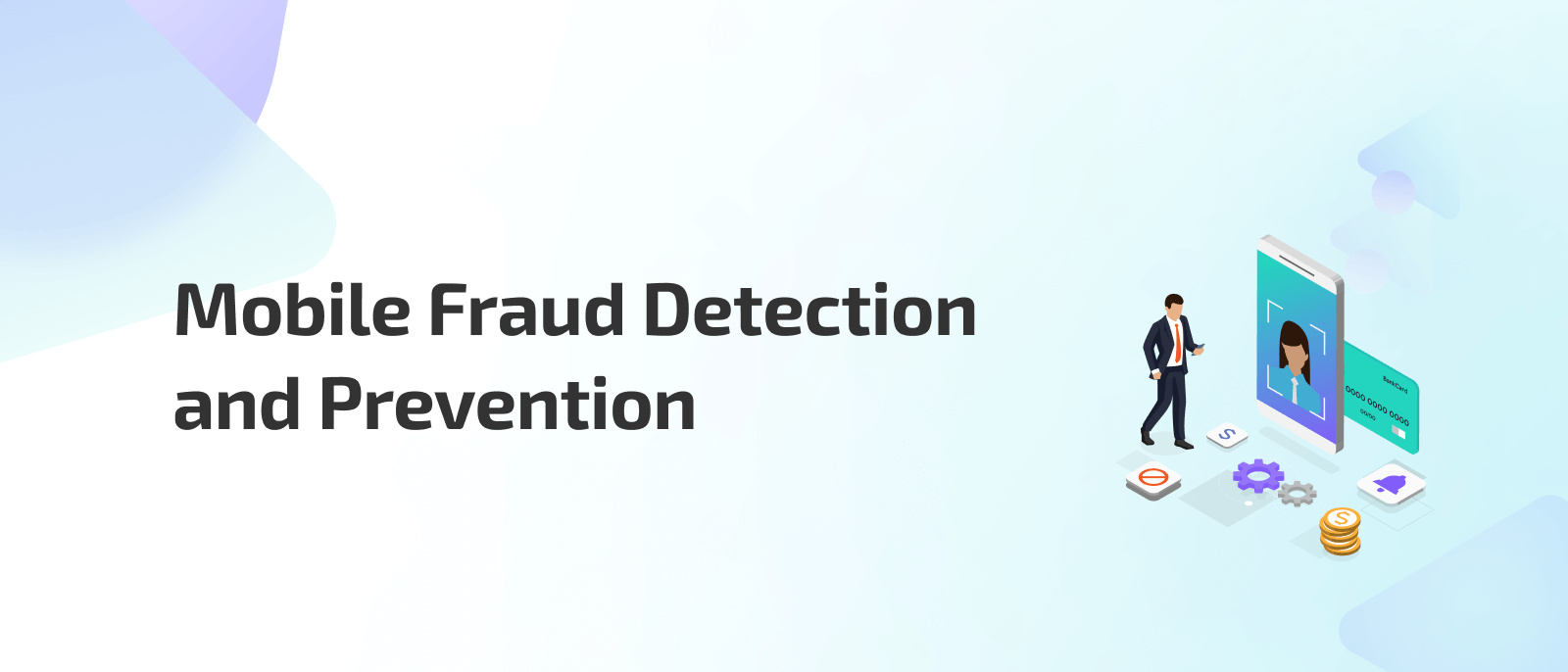
Accelerate AML Compliance: Meet Regulatory Demands with 80% Less Setup Time
In the rapidly evolving landscape of mobile applications and digital transactions, mobile fraud has emerged as a significant threat to businesses and consumers alike. As more people rely on mobile devices for banking, including the need for online banking fraud solutions, shopping, and various other activities, the importance of robust mobile fraud detection and prevention mechanisms cannot be overstated. In this comprehensive article, we will explore the intricacies of mobile fraud, the techniques used for its detection, and the best practices to safeguard your business against fraudulent activities.
What is Mobile Fraud Detection?
Mobile fraud refers to the unauthorized or malicious use of mobile devices, applications, or services for financial gain or other illegal purposes. It encompasses a wide range of activities, including account takeovers, click fraud, mobile app install fraud, SMS fraud, and in-app purchase fraud. The prevalence of mobile fraud has risen in tandem with the increasing adoption of mobile technologies, making it a critical concern for businesses operating in the digital realm.
Comply quickly with local/global regulations with 80% less setup time
Types of Mobile Fraud
1. Account Takeover Fraud
Account takeover fraud occurs when fraudsters gain unauthorized access to a user's account by exploiting stolen credentials or other means. This type of fraud can lead to financial losses, data breaches, and damage to a company's reputation.
2. Click Fraud
Click fraud involves deliberately generating invalid or fraudulent clicks on online advertisements, typically through bots or click farms, highlighting the importance of fraudulent application protection. This practice can inflate advertising costs and skew campaign performance metrics.
3. Mobile App Install Fraud
Mobile app install fraud involves the artificial inflation of app installation numbers by using automated systems or incentivized users. This type of fraud can mislead advertisers and app developers, resulting in inaccurate performance data and wasted marketing budgets.
4. SMS Fraud
SMS fraud involves the unauthorized or malicious use of SMS services, such as sending unsolicited or fraudulent messages or exploiting premium SMS services for financial gain.
5. In-app Purchase Fraud
In-app purchase fraud occurs when fraudsters exploit vulnerabilities in mobile applications to obtain digital goods or services without proper payment, making mobile app fraud detection crucial. This type of fraud can lead to significant revenue losses for app developers and publishers.
Fraud Detection Techniques
Effective mobile fraud detection solution relies on a combination of techniques, including rule-based approaches, machine learning algorithms, and hybrid models.
1. Rule-based Fraud Detection
Rule-based fraud detection systems rely on predefined rules and patterns based on known fraud scenarios. These rules can be manually defined by experts or derived from historical data analysis. While rule-based systems are relatively straightforward to implement, they may struggle to detect new or evolving fraud patterns.
2. Machine Learning-based Fraud Detection
Machine learning techniques, including supervised and unsupervised learning algorithms, have gained significant traction in mobile fraud detection due to their ability to learn and adapt from data.
3. Supervised Learning Techniques
Supervised learning algorithms, such as logistic regression, decision trees, and random forests, are trained on labeled data (known instances of fraud and non-fraud) to learn patterns and predict new, unseen data.
4. Unsupervised Learning Techniques
Unsupervised learning techniques, including clustering and anomaly detection, do not require labeled data. These algorithms identify patterns and anomalies in the data, which can be useful for detecting previously unknown fraud scenarios.
5. Deep Learning Techniques
Deep learning techniques, such as artificial neural networks and autoencoders, have shown promising results in mobile fraud detection. These algorithms can learn complex patterns and relationships from large volumes of data, making them well-suited for detecting sophisticated fraud scenarios.
6. Hybrid Approaches
Hybrid approaches combine rule-based systems and machine learning techniques, leveraging the strengths of both methodologies. These hybrid models can benefit from the interpretability and domain expertise of rule-based systems while harnessing the adaptability and pattern recognition capabilities of machine learning algorithms.
Data Sources for Fraud Detection
Effective mobile fraud detection relies on diverse data sources, including:
- Device data: Information such as device fingerprinting, sensor data, and hardware specifications can provide valuable insights into potential fraud scenarios.
- User behavior data: Monitoring user behavior patterns, app usage, and location data can help identify anomalous activities that may indicate fraud.
- Transaction data: Analyzing transaction details, including amounts, frequencies, and patterns, can aid in detecting suspicious activities.
- Network data: Monitoring network traffic and IP addresses can help identify coordinated fraud attempts or bot activities.
- External data sources: Leveraging third-party data sources, such as IP reputation databases or threat intelligence feeds, can enhance the overall effectiveness of fraud detection systems.
Challenges in Mobile Fraud Detection
While mobile fraud detection techniques continue to evolve, several challenges persist:
1. Data Quality and Availability
Ensuring high-quality and comprehensive data is crucial for effective fraud detection. However, data quality issues, such as incomplete or inaccurate data, can hinder the performance of fraud detection systems.
2. Evolving Fraud Patterns
As fraudsters continuously adapt their tactics, fraud detection systems must be able to identify and respond to new and evolving fraud patterns promptly.
3. Privacy and Data Protection Concerns
Collecting and processing user data for mobile fraud protection solutions raises privacy and data protection concerns. Businesses must balance effective fraud detection and compliance with relevant regulations and user privacy expectations.
4. Real-time Fraud Detection
In the fast-paced mobile environment, detecting and responding to fraud in real-time is essential. Delays in fraud detection can lead to significant financial losses and damage a business's reputation.
Best Practices and Future Trends
To effectively combat mobile fraud, businesses should adopt the following best practices and stay informed about emerging trends:
1. Continuous Monitoring and Adaptation
Monitoring fraud patterns and adapting detection systems to emerging threats is crucial. Regular updates and refinements to models and rules can help maintain the effectiveness of fraud detection mechanisms.
2. Multi-factor Authentication
Implementing robust multi-factor authentication techniques, such as biometrics or one-time passwords, can significantly enhance account security and reduce the risk of account takeover fraud.
3. Emerging Technologies
Emerging technologies, such as blockchain and federated learning, hold promise for enhancing mobile fraud detection capabilities. Blockchain can provide secure and transparent transaction records, while federated learning enables collaborative model training without compromising data privacy.
According to a report by Juniper Research, the global cost of online fraud is expected to reach $48 billion by 2023, with a significant portion attributed to mobile fraud.
The staggering projected cost underscores the urgent need for businesses to adopt advanced mobile fraud prevention measures to protect their operations and customers.
FOCAL Solution: AI-Powered Fraud Prevention Software
FOCAL offers an AI-powered fraud prevention solution designed to stay ahead of fraudsters and protect businesses from sophisticated attacks. Their solution provides the following key features:
1. Combat Sophisticated Attacks
FOCAL advanced fraud prevention software employs cutting-edge machine learning algorithms and deep learning techniques to detect and prevent even the most sophisticated fraud attempts, including account takeovers, click fraud, and in-app purchase fraud.
2. Proactive Defense
FOCAL solution takes a proactive approach to fraud prevention, continuously monitoring user behavior, transaction patterns, and other relevant data sources to identify potential threats before they can cause significant damage.
3. Real-time Detection
With real-time fraud detection capabilities, FOCAL solution can swiftly identify and respond to fraudulent activities as they occur, minimizing the risk of financial losses and reputational damage.
4. Identifying Non-Transactional Fraud
Beyond traditional transactional fraud, FOCAL solution can detect and mitigate non-transactional fraud, such as account takeovers, identity theft, and other malicious activities that may not involve direct financial transactions.
5. Plug and Play Fraud Prevention
FOCAL fraud prevention solution is designed for seamless integration with existing systems and workflows, enabling businesses to quickly deploy and benefit from its advanced fraud detection capabilities without disrupting their operations.
Conclusion
In the rapidly evolving mobile landscape, effective mobile fraud detection and prevention are crucial for safeguarding businesses and protecting customer trust. By leveraging a combination of rule-based systems, machine learning techniques, and robust data sources, organizations can stay ahead of fraudsters and mitigate mobile fraud risks.
As mobile technologies advance and fraud tactics become more sophisticated, businesses must remain vigilant and adopt a proactive approach to fraud detection. Continuous monitoring, adaptation, and collaboration with industry partners and solution providers like FOCAL will be essential in maintaining a strong defense against mobile fraud.
Looking ahead, the integration of emerging technologies, such as blockchain and federated learning, holds promise for enhancing the effectiveness and privacy-preserving capabilities of mobile fraud detection systems. Additionally, ongoing research and innovation in areas like deep learning and explainable AI will further strengthen the ability to detect and prevent mobile fraud while maintaining transparency and trustworthiness.
By prioritizing mobile fraud detection and prevention, businesses can foster a secure and trustworthy digital ecosystem, enabling seamless and safe mobile experiences for their customers.
Streamline Compliance: Achieve 80% Faster Setup for Fraud Prevention

How Aseel reduced onboarding time by more than 87% using FOCAL
Learn how FOCAL empowered Aseel to achieve new milestones.
Mastering Fraud Prevention: A Comprehensive Guide for KSA and MENA Businesses
51% of organizations fell victim to fraud in the last two years, don't be caught off guard, act proactively.
Featured blog posts
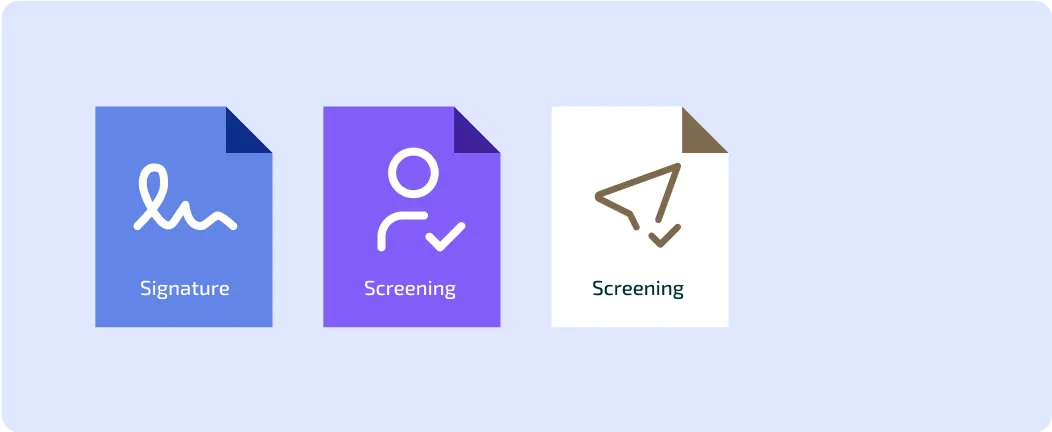
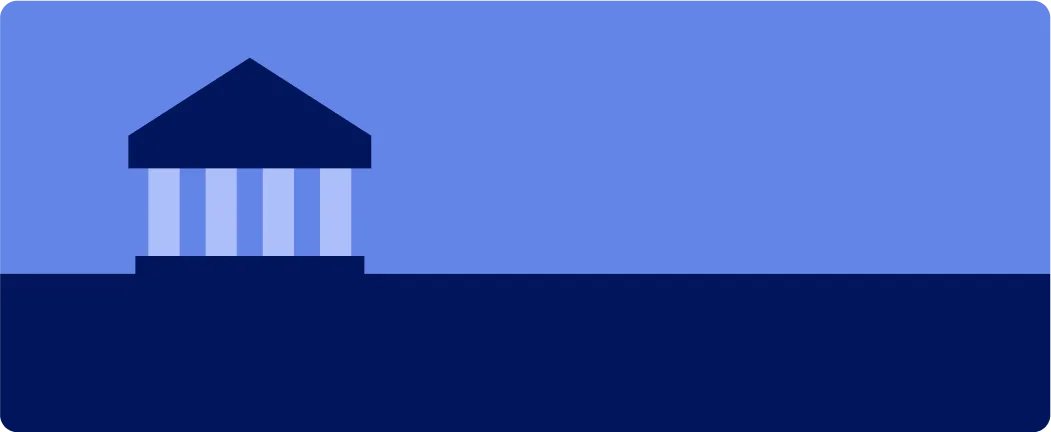

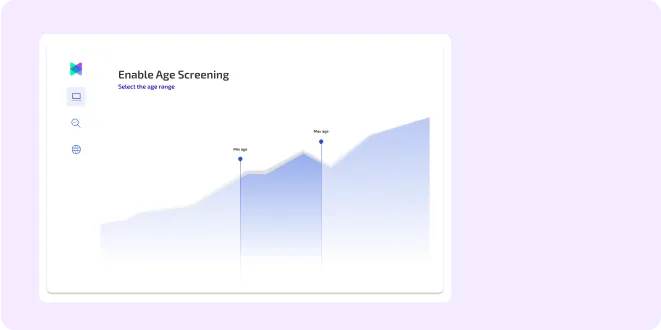
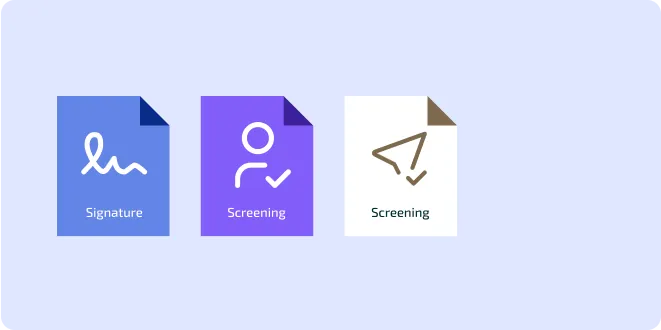
Comments
Leave a Reply
Comment policy: We love comments and appreciate the time that readers spend to share ideas and give feedback. However, all comments are manually moderated and those deemed to be spam or solely promotional will be deleted.