.webp)
Published on
December 30, 2024
Fraud Detection with Machine Learning & Artificial Intelligence in 2025
In this story
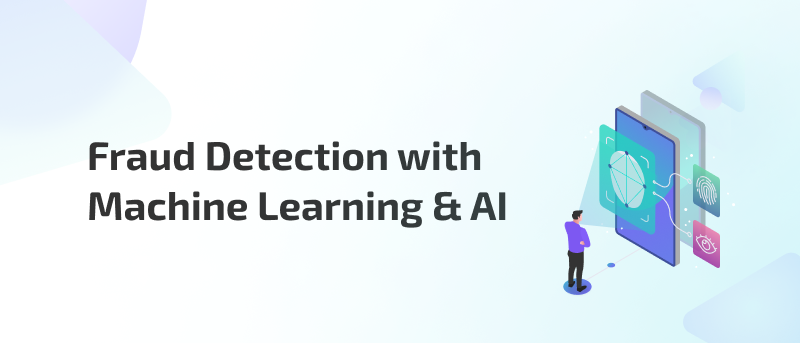
Accelerate AML Compliance: Meet Regulatory Demands with 80% Less Setup Time
In the ever-evolving landscape of cybersecurity, adopting a proactive stance is paramount. To protect your company against cybercriminals, you must understand their tactics. AI for fraud detection emerges as the strategic ally in this battle. It offers dynamic capabilities to counteract the constant evolution of malicious techniques.
Fraud detection using machine learning is another powerful tool in this arsenal, providing advanced capabilities to identify and prevent fraudulent activities. Fraud detection with machine learning is becoming increasingly essential in the face of sophisticated cyber threats. Attackers use advanced scripts and exploit vulnerabilities. This makes the need for strong defenses more apparent than ever.
In this article, we will delve into what machine learning (ML) and artificial intelligence (AI) are. We will discuss their types and how they differ from each other. We will also explore how ML and AI can benefit financial institutions in detecting and preventing fraud.
What is Artificial Intelligence?
Artificial Intelligence, often abbreviated as AI, refers to the development of computer systems. They can perform tasks that typically require human intelligence. These tasks include learning from experience and understanding natural language. They also involve recognizing patterns, solving problems, and making decisions.
AI aims to create machines capable of simulating human intelligence and performing tasks autonomously. AI fraud detection plays a critical role in securing systems against fraudulent activities.
Types of Artificial Intelligence
1. Narrow or Weak AI
This type of AI is designed for a specific task and operates within a limited context. It excels at performing a defined set of functions but lacks the broad cognitive abilities associated with human intelligence.
Narrow AI could be virtual personal assistants and recommendation systems. AI in fraud prevention contributes to the development of strong defenses against specific fraudulent activities.
2. General or Strong AI
General AI refers to a system with the ability to understand, learn, and apply knowledge across various domains. It is similar to human intelligence. Achieving strong AI is a complex challenge and remains an area of ongoing research and development. Artificial intelligence for fraud detection contributes to the development of strong defenses against specific fraudulent activities.
AI systems use various techniques. These include machine learning, natural language processing, computer vision, and robotics. Machine learning is a subset of AI. It involves training algorithms with data. This enables them to improve their performance over time without explicit programming. Machine learning fraud detection and artificial intelligence for fraud detection leverage advanced algorithms to adapt to evolving cyber threats.
The applications of AI are diverse, spanning industries such as healthcare, finance, gaming, education, and entertainment. They come in different forms, for instance, autonomous vehicles, language translation, image and speech recognition, and advanced data analysis. AI in financial fraud detection and AI for fraud detection examples highlight the versatile applications of AI in securing various sectors against fraudulent activities.
In essence, AI represents the frontier of computer science. It aims to create intelligent systems that can adapt, learn, and perform tasks traditionally linked to human intelligence. Fraud detection AI examples showcase the practical applications of AI in combating fraudulent activities.
What is Machine Learning?
Machine learning is a subset of artificial intelligence (AI). It focuses on developing algorithms and models that enable computers to learn from data. Machine learning allows systems to identify patterns, make predictions, and improve their performance over time through experience. It does not rely on explicit programming. Fraud detection with machine learning is particularly effective due to its ability to adapt and evolve based on new data. Fraud detection using machine learning enhances the capabilities of systems to recognize and respond to fraudulent patterns.
The process involves feeding large amounts of data into the system. The machine uses this data to identify and learn patterns. This enables the machine to make informed decisions and predictions without being explicitly programmed for each task. The three main types of machine learning are supervised learning, unsupervised learning, and reinforcement learning.
Types of Machine Learning
Machine learning encompasses three primary types, each serving distinct learning purposes. Machine learning for fraud detection leverages these types to enhance the efficiency of fraud prevention systems.
1. Supervised Learning
Supervised learning mirrors a teacher-student dynamic. The computer algorithm is provided with a dataset containing both input data (problems) and corresponding correct output (answers). The algorithm studies the dataset, discerns patterns, and can then predict or decide outcomes for new, unseen data. It's like a student learning from examples. Machine learning fraud detection, in this context, relies on supervised learning to recognize and respond to known patterns of fraudulent behavior.
2. Unsupervised Learning
Unsupervised learning adopts a detective's approach, seeking patterns or structures without explicit examples. The algorithm delves into a dataset containing only input data, devoid of corresponding correct outputs. It analyzes the data autonomously, uncovering hidden patterns or relationships.
This method is akin to solving a case with no initial leads, relying on clues and connections within the available information. Machine learning for fraud detection uses unsupervised learning to identify novel patterns indicative of potential fraudulent activities.
3. Reinforcement Learning
Reinforcement learning draws parallels with training a dog to perform tricks. The computer algorithm, acting as an agent, interacts with an environment and receives feedback in the form of rewards or penalties for each decision made.
The algorithm's objective is to learn the optimal strategy or policy, maximizing cumulative rewards over time. Through trial and error, the algorithm refines its strategy based on the received feedback. Machine learning and fraud detection combine forces through reinforcement learning to continually adapt to evolving cyber threats.
The combination of AI and machine learning for fraud detection provides a multifaceted defense against cyber threats. AI for fraud prevention and detection, along with machine learning, empowers organizations to stay ahead in the ongoing battle against cybercriminals.
Fraud detection using AI in banking and AI in financial fraud detection further emphasizes the widespread adoption of these technologies across various sectors to ensure strong cybersecurity.
Comply quickly with local/global regulations with 80% less setup time
The Role of ML and AI in Fraud Detection
Financial institutions (FIs) integrate Machine Learning (ML) and Artificial Intelligence (AI) into fraud detection for enhanced accuracy and efficiency. As outlined in a whitepaper authored by computer scientists at the University of Jakarta, fraud detection machine learning algorithms demonstrated a remarkable 96% accuracy in minimizing fraud for eCommerce enterprises.
ML algorithms analyze vast datasets, recognizing patterns and anomalies indicative of fraudulent activities. AI, particularly in the form of deep learning, excels in discerning complex, evolving fraud patterns. These technologies enable real-time monitoring, adaptability to new threats, and proactive prevention, which is crucial for safeguarding financial transactions in the face of sophisticated and dynamic fraudulent activities.
The combination of fraud detection machine learning and AI empowers FIs to stay ahead in the constant cat-and-mouse game with fraudsters, providing a strong defense against diverse fraud types.
- Enhanced Accuracy: Fraud detection machine learning and AI models can analyze large datasets and identify subtle patterns. This significantly improves fraud detection accuracy compared to traditional methods.
- Real-time Monitoring: Fraud detection machine learning and AI enable real-time monitoring of transactions. They help financial institutions detect and respond to suspicious activities promptly, reducing the potential impact of fraud.
- Adaptability to Evolving Patterns: These technologies can adapt to new and evolving fraud patterns. They provide a proactive defense against emerging threats without needing constant manual updates.
- Efficient Data Processing: Fraud detection machine learning algorithms efficiently process vast amounts of data, automating the analysis of transactions and reducing the time and resources required for fraud detection.
- Reduced False Positives: Fraud detection machine learning and AI models can learn from historical data, minimizing false positives and enhancing the precision of fraud detection, thereby improving the overall efficiency of fraud prevention systems.
- Advanced Anomaly Detection: Fraud detection machine learning excels at identifying anomalies in data, making it effective in detecting irregular patterns that might indicate fraudulent activities, even if those patterns are not explicitly defined.
- Cost Savings: Automated fraud detection through fraud detection machine learning and AI can lead to cost savings for financial institutions by reducing the need for extensive manual reviews and investigations.
- Improved Customer Experience: By minimizing false positives and preventing genuine transactions from being flagged as fraudulent, fraud detection machine learning and AI contribute to a smoother and less intrusive customer experience.
- Scalability: Fraud detection machine learning and AI systems can scale to handle large and growing volumes of transactions, making them suitable for the dynamic and expanding nature of financial activities.
- Enhanced Security Posture: Fraud detection machine learning and AI contribute to an institution's overall security posture by continuously learning and adapting, providing a proactive defense against the evolving tactics employed by fraudsters.
10 Common Applications of Machine Learning in Fraud Detection
Financial institutions can use fraud detection machine learning for advanced fraud detection and prevention in various scenarios.
Financial institutions elevate their defenses against many fraudulent activities by harnessing the power of fraud detection machine learning. This strategic integration ensures the safeguarding of customer accounts. It also upholds regulatory compliance and minimizes financial losses.
Below are the ten most popular use cases where financial institutions leverage fraud detection machine learning for advanced fraud detection and prevention.
1. Credit Card Fraud Detection
Fraud detection machine learning algorithms analyze transaction data. They consider factors like time, location, and spending patterns. This helps them identify anomalies in real-time. Unusual activities, such as transactions from distant locations or large transactions deviating from the norm, can trigger alerts for potential credit card fraud.
2. ATM and Point-of-Sale (POS) Fraud Prevention
Fraud detection machine learning models can monitor ATM transactions and POS activities. They can detect irregularities like multiple cash withdrawals in a short time or transactions outside regular customer behavior patterns. These irregularities may indicate fraudulent activities.
3. Identity Verification
Fraud detection machine learning contributes to strong identity verification by analyzing various data points, including biometric information, transaction history, and user behavior, ensuring that only authorized individuals access financial services and preventing identity theft.
4. Loan Application Fraud Detection
Fraud detection machine learning algorithms assess loan applications by analyzing a multitude of factors, including credit history, income, and application details. This aids in identifying potentially fraudulent loan applications or instances of identity theft.
5. Wire Transfer and Transaction Monitoring
Fraud detection machine learning is instrumental in monitoring wire transfers and transactions, identifying unusual patterns, and flagging potential instances of money laundering or fraudulent fund transfers.
6. Account Takeover Prevention
Fraud detection machine learning models continuously analyze user login behavior, detecting anomalies such as multiple failed login attempts, access from unfamiliar devices, or unusual account activity, thus thwarting potential account takeovers.
7. Insider Fraud Detection
Fraud detection machine learning can help detect insider fraud by analyzing employee behavior and identifying unusual patterns in access to sensitive information or transactions that deviate from normal employee activity.
8. Automated Fraud Reporting and Investigation
Fraud detection machine learning tools streamline fraud reporting and investigation processes by automating the analysis of vast datasets, helping investigators focus on high-priority cases and reducing response times.
9. Customer Communication Analysis
Fraud detection machine learning can analyze customer communications, such as emails or chat messages, to detect phishing attempts, fraudulent solicitations, or other forms of social engineering targeting customers.
10. Regulatory Compliance
Fraud detection machine learning assists FIs in maintaining regulatory compliance by automating the monitoring of financial transactions, identifying suspicious activities, and generating reports required for compliance reporting.
Fraud Detection with FOCAL
FOCAL is revolutionizing fraud detection for businesses. FOCAL fraud prevention suite departs from manual and reactive approaches. It ensures real-time identification of fraud, enabling prompt and proactive interventions.
FOCAL employs machine learning and artificial intelligence to scrutinize data from diverse sources, recognizing suspicious patterns and behaviors indicative of fraudulent activities. Continuously learning and adapting, FOCAL remains a step ahead of fraudsters by detecting emerging fraud trends. FOCAL also significantly reduces false positives, mitigating investigation costs and potential impacts on customer relationships.
With its advanced algorithms, FOCAL precisely distinguishes legitimate transactions. It stands as a formidable tool for organizations seeking to enhance their fraud detection and prevention capabilities, offering real-time detection, continuous learning, and minimal false positives.
Conclusion
In conclusion, the integration of AI and machine learning is a crucial element within a comprehensive risk management strategy. This article has illuminated the advantages and considerations linked to utilizing machine learning in fraud prevention. It empowers businesses to strengthen their defenses against continually evolving cyber threats.
Organizations can proactively fortify their security measures by comprehending the intricacies of this technology. In a landscape where adaptability is paramount, incorporating AI and ML into fraud detection and prevention strategies is proactive and indispensable. It helps preserve the digital integrity of businesses.
FAQs:
Q1. Can Artificial Intelligence And Machine Learning Be Used Interchangeably?
No. Artificial Intelligence (AI) and Machine Learning (ML) are related but not interchangeable. Instead, they share a symbiotic relationship within computer science.
Artificial Intelligence (AI) and Machine Learning (ML) are related concepts but not interchangeable. AI is the broader field focused on creating intelligent machines, while ML is a specific subset within AI, emphasizing the ability of systems to learn and improve from data without explicit programming. ML is a tool within the realm of AI, contributing to its diverse capabilities.
Q2. How Outsourced vs On-Site ML for Fraud Detection Differ in Data Control and Management
Outsourced machine learning for fraud detection involves third-party providers managing the process off-site. This offers convenience but may sacrifice data control. On-site solutions keep operations in-house, providing greater data control. However, they require substantial infrastructure and expertise investments.
Q3. Is Fraud Detection with Machine Learning Expensive?
Costs may vary. However, the investment in machine learning for fraud detection is often justified. This is due to reduced financial losses and improved security measures.
Q4. Can Machine Learning Prevent Friendly Fraud?
Yes. Machine learning can identify patterns related to friendly fraud. It analyzes customer purchase history, return rates, and chargeback patterns.
Streamline Compliance: Achieve 80% Faster Setup for Fraud Prevention

How Aseel reduced onboarding time by more than 87% using FOCAL
Learn how FOCAL empowered Aseel to achieve new milestones.
Mastering Fraud Prevention: A Comprehensive Guide for KSA and MENA Businesses
51% of organizations fell victim to fraud in the last two years, don't be caught off guard, act proactively.
Featured blog posts
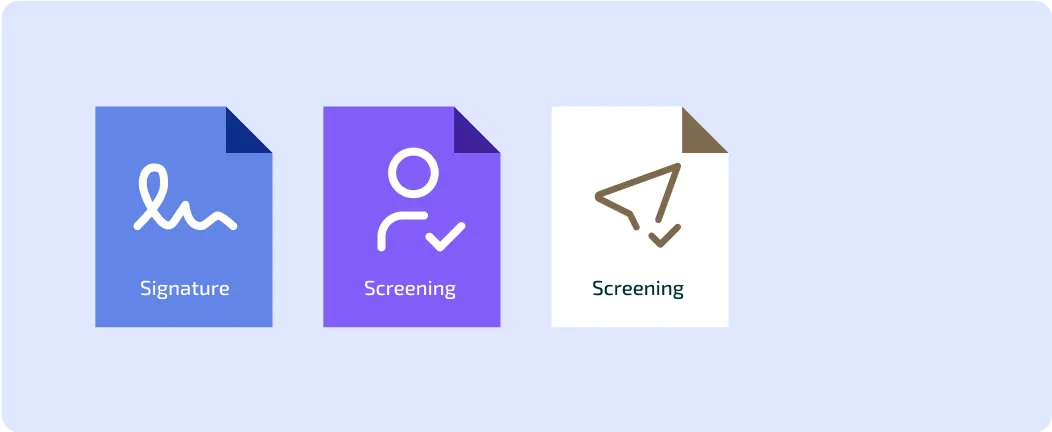
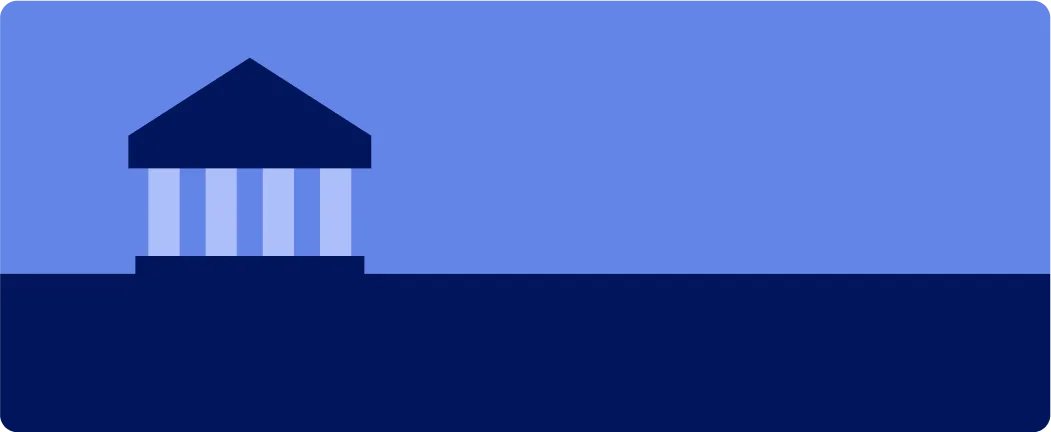

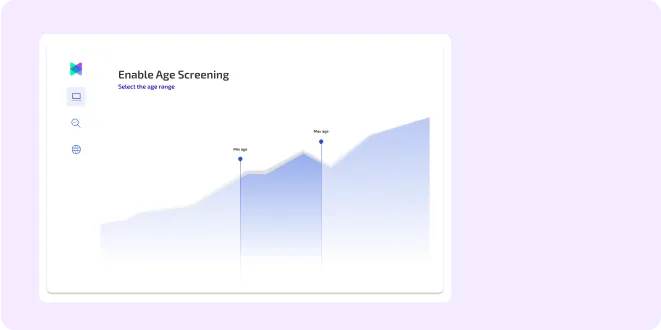
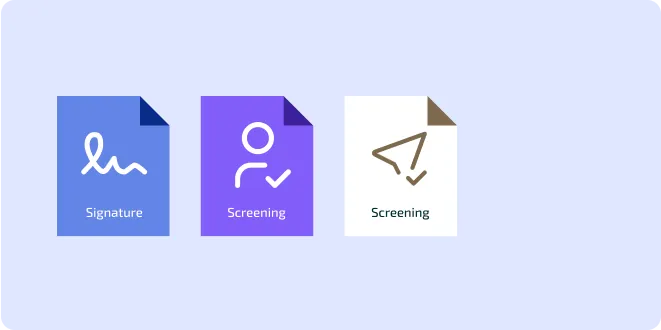
Comments
Leave a Reply
Comment policy: We love comments and appreciate the time that readers spend to share ideas and give feedback. However, all comments are manually moderated and those deemed to be spam or solely promotional will be deleted.