.webp)
Published on
December 30, 2024
Fraud Analytics: Identifying and Reducing Fraud Risks in 2025
In this story

Accelerate AML Compliance: Meet Regulatory Demands with 80% Less Setup Time
Fraud analytics has become crucial to business security, especially as more transactions are conducted online. As we move through 2025, it's essential to grasp the nuances of fraud analytics and its critical role in safeguarding businesses.
What is Fraud Analytics?
Fraud analytics involves using data analysis tools to detect and prevent fraud. This process includes examining data from various sources, spotting inconsistencies, and identifying patterns that may indicate fraudulent activities. The application of fraud analytics is not confined to one industry but is a crucial component across various sectors, especially in banking and financial services.
The Importance of Fraud Analytics for Your Business
Fraud analytics is vital for businesses as it aids in minimizing financial losses, maintaining customer trust, and ensuring regulatory compliance. It's not just a safety measure but also a strategic approach for staying competitive. Additional key aspects include:
- Minimizing Financial Losses: Early detection of fraud to save substantial amounts.
- Maintaining Customer Trust: Securing transactions to boost customer confidence.
- Regulatory Compliance: Essential for adhering to legal standards.
- Risk Assessment: Identifies unforeseen risks through data analysis.
- Resource Allocation: Efficiently allocating resources where they're needed most.
- Strategy Improvement: Enhances existing risk strategies through continuous monitoring.
- Repeatability in Business Expansion: Provides a framework for launching new products or entering new markets.
- Streamlining Workload: Makes risk management teams more efficient with fraud analytics tools.
Incorporating fraud analytics is not just a safety measure but a strategic move to maintain a competitive edge in the market.
Using Data Analytics for Fraud Detection
Data analytics for fraud detection is a systematic approach involving several key steps:
- Data Collection: Gathering relevant data from various sources.
- Analysis: Applying algorithms to analyze patterns and behaviors.
- Detection: Identifying anomalies that may indicate fraudulent activities.
- Action: Implementing measures to prevent fraud based on findings.
- Endpoint-Centric Analysis: This examines user devices and registration information for irregularities, like a new device suddenly accessing an established account.
- Navigation/Behavior-Centric Analysis: This involves analyzing how users interact with services, looking for unusual patterns that deviate from normal user behavior.
- Account-Centric Analysis: This focuses on detecting suspicious activities within specific accounts, such as irregular transaction patterns.
- Cross-Channel Analysis: This compares user behavior across different platforms or channels, identifying inconsistencies that suggest fraudulent behavior.
- Entity Linking Analysis: This is a deeper examination of how different entities (like accounts, devices, and IP addresses) are connected, revealing complex fraud networks.
Utilizing data effectively is crucial in detecting and preventing fraudulent activities. For more insights, explore our Fraud Prevention Strategies.
Comply quickly with local/global regulations with 80% less setup time
Fighting Financial Fraud with Big Data Analytics
Big data has revolutionized fraud detection analytics. By analyzing vast datasets, businesses can now uncover sophisticated fraud schemes that were previously undetectable. Essential techniques include:
- Data Collection and Integration: This is the first and crucial step. Financial institutions collect vast amounts of data from various sources, including transaction records, customer information, and interaction data. Big data technologies enable the integration of this data from diverse sources, ensuring a comprehensive dataset for analysis.
- Data Processing and Management: Big data analytics requires handling large volumes of data, which often includes unstructured data. Techniques like data mining and preprocessing are used to clean, normalize, and structure this data for effective analysis.
- Anomaly Detection: One of the primary techniques in combating financial fraud is anomaly detection. Using algorithms, the system can identify unusual patterns or transactions that deviate from the norm. These anomalies could indicate potential fraudulent activities. Machine learning models, especially those involving unsupervised learning, are often used for this purpose.
- Predictive Analytics: This involves using statistical models and machine learning algorithms to predict the likelihood of fraudulent activities. By analyzing historical data, these models can identify patterns and trends associated with fraud and use this information to predict and flag potential future frauds.
- Network Analysis: Financial fraud often involves complex relationships and networks (like money laundering schemes). Big data analytics can use network analysis techniques to uncover hidden connections and patterns among transactions and accounts that might indicate fraudulent networks.
- Real-time Monitoring and Surveillance: Big data enables the real-time analysis of transactions and activities. This is crucial for timely fraud detection, allowing institutions to react quickly to prevent or minimize losses.
- Regulatory Compliance and Reporting: Big data analytics also helps financial institutions comply with regulatory requirements. By analyzing transactional data, they can ensure adherence to laws and regulations regarding financial practices and report suspicious activities to authorities.
- Continuous Learning and Adaptation: As fraudsters continually change their tactics, the analytical models must also evolve. Machine learning models are trained continuously with new data, ensuring that the detection mechanisms stay effective against evolving fraud strategies.
- User Behavior Analytics (UBA): This technique analyzes user behavior to detect anomalies. For instance, if a user's transaction pattern changes significantly, it could signal potential fraud.
- Risk Assessment: Big data analytics also helps assess the risk level of transactions or clients based on historical data, helping make informed decisions on whether to flag transactions as fraudulent.
- Integration with Other Systems: Finally, the insights gained from big data analytics need to be integrated with other systems like customer relationship management (CRM) and enterprise resource planning (ERP) to ensure a holistic approach to fraud prevention.
Combating financial fraud with big data analytics involves a multi-faceted approach that includes collecting and processing large datasets, applying advanced analytical techniques like machine learning for anomaly detection and predictive analytics, and continuously adapting to new patterns of fraudulent activities.
Predictive Analytics: A Proactive Stance Against Fraud
One of the major challenges faced by businesses and financial institutions is the constant threat of fraud. Fraudulent activities are becoming more sophisticated. Traditional fraud detection methods are proving insufficient. In this landscape, fraud analysis is a powerful tool. It offers a proactive stance against fraud.
1. Understanding Predictive Analytics
Predictive analytics uses statistical algorithms, machine learning, and data mining to analyze historical data. It identifies patterns that predict future events. In fraud prevention, fraud analytics in banking uses vast data to identify subtle patterns that suggest fraud. This method goes beyond traditional rule-based systems. It allows organizations to stay one step ahead of fraudsters.
2. The Role of Big Data
The advent of big data has revolutionized the way organizations approach fraud prevention. Predictive analytics harnesses the power of big data by analyzing massive datasets in real time. This enables organizations to identify anomalies and detect potentially fraudulent transactions or activities as they occur. The ability to process and analyze large volumes of data swiftly is crucial in staying ahead of the ever-evolving nature of fraud.
3. Machine Learning Algorithms
Machine learning algorithms play a pivotal role in predictive analytics for fraud prevention. These algorithms learn from historical data and adapt to new patterns, continuously improving their accuracy over time. By employing machine learning, organizations can develop models that can detect emerging fraud trends and adjust their strategies accordingly. This adaptability is essential in combating fraud schemes that evolve rapidly.
4. Behavioral Analysis
Predictive analytics goes beyond traditional methods by incorporating fraud data analytics. It considers not only individual transactions but also the overall behavior of users. Predictive analytics can quickly identify deviations that may indicate fraudulent activity by understanding patterns of normal behavior. This approach enables a proactive response to potential threats before they escalate.
5. Real-Time Fraud Detection
One of the key advantages of predictive analytics is its ability to provide real-time fraud detection. Traditional methods often involve retrospective analysis, meaning fraudulent activities are identified after they have occurred. Predictive analytics, however, allows organizations to intervene in real-time, preventing fraudulent transactions before they are completed.
4 Steps to Deploying a Fraud Analytics Solution
Deploying a fraud analytics solution is a structured process involving:
1. Data Integration: Merging Data from Various Sources
- Gathering Diverse Data Sets: This step involves collecting data from a variety of sources, such as transaction records, customer profiles, and operational data. This may include both structured data (like databases) and unstructured data (like emails or social media posts).
- Ensuring Data Quality: Ensuring the integrity and accuracy of the data is crucial. This involves cleaning the data, handling missing values, and ensuring consistency across different data sets.
- Data Consolidation: Integrating these diverse data sets into a single, cohesive database. This may involve data transformation processes to ensure compatibility and effective integration.
2. Model Development: Creating Algorithms Based on Business Specifics
- Understanding Business Requirements: Tailoring the fraud detection models to suit the specific needs and risk profiles of the business.
- Selection of Algorithms: Choosing appropriate machine learning algorithms (like decision trees, neural networks, or anomaly detection algorithms) based on the nature of the data and the specific types of fraud likely to be encountered.
- Training and Testing Models: Developing models using historical data and continually testing and refining them to improve accuracy and reduce false positives.
3. Continuous Monitoring: Ongoing System Updates for Detecting New Fraud Types
- Real-time Analysis: Implementing systems that can analyze transactions in real time, allowing for immediate detection of potential fraud.
- Adapting to New Patterns: As fraudsters continually evolve their tactics, the system must be capable of learning new patterns of fraudulent behavior. This involves regular updates to the model based on new data and trends.
- System Health Checks: Regularly assessing the performance of the fraud detection system to ensure it's functioning as intended.
4. Feedback Loop: Adjusting Strategies Based on Effectiveness
- Performance Evaluation: Analyzing the effectiveness of the fraud detection models by looking at metrics such as the rate of successful fraud detection, false positives, and false negatives.
- Incorporating Feedback: Using insights gained from the performance evaluation to refine and adjust the models. This might involve retraining the models with new data or tweaking the algorithms.
- Stakeholder Engagement: Ensuring continuous communication with stakeholders (like risk management teams, IT staff, and executives) to align the fraud detection strategies with business objectives and risk management policies.
Deploying a fraud analytics solution is a dynamic and iterative process that requires careful attention to data integration, tailored model development, continuous monitoring for new fraud types, and a strong feedback mechanism to ensure ongoing effectiveness and relevance in a rapidly evolving landscape.
FOCAL's: Enhancing Your Fraud Prevention Capabilities
FOCAL's offers advanced solutions in fraud prevention, combining real-time monitoring with sophisticated analytics. Their approach is designed to combat various types of fraud effectively, including customer screening against global sanction lists and PEP lists, risk scoring based on customer profiles, transaction monitoring, and automated workflows.
FOCAL is designed for emerging markets and helps businesses swiftly comply with global and local Anti-Fraud regulations while simplifying complex processes. It's trusted by leading financial institutions for its efficiency and adaptability to specific industry needs. Learn how FOCAL can assist in your anti-fraud efforts.
Conclusion
As we wrap up our guide, it's clear that fraud analytics is crucial in protecting our financial and digital assets now and in the years to come. This guide aims to give you the tools and knowledge to understand and implement effective fraud prevention strategies, including conducting thorough fraud investigations.
Looking ahead, the fight against fraud will continue to evolve. New technologies and methods will emerge, and fraudsters will keep finding new ways to challenge our systems. This means we must be alert, flexible, and ready to adapt to new threats as they arise.
Streamline Compliance: Achieve 80% Faster Setup for Fraud Prevention

How Aseel reduced onboarding time by more than 87% using FOCAL
Learn how FOCAL empowered Aseel to achieve new milestones.
Mastering Fraud Prevention: A Comprehensive Guide for KSA and MENA Businesses
51% of organizations fell victim to fraud in the last two years, don't be caught off guard, act proactively.
Featured blog posts
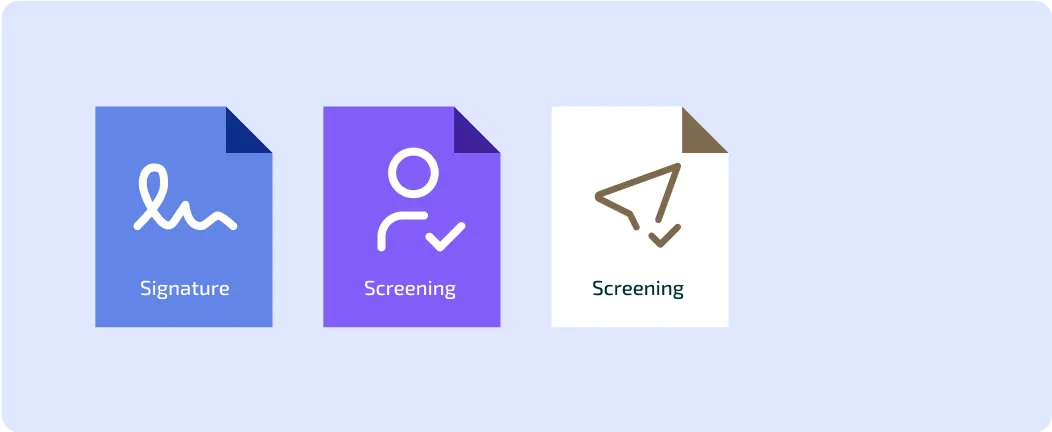
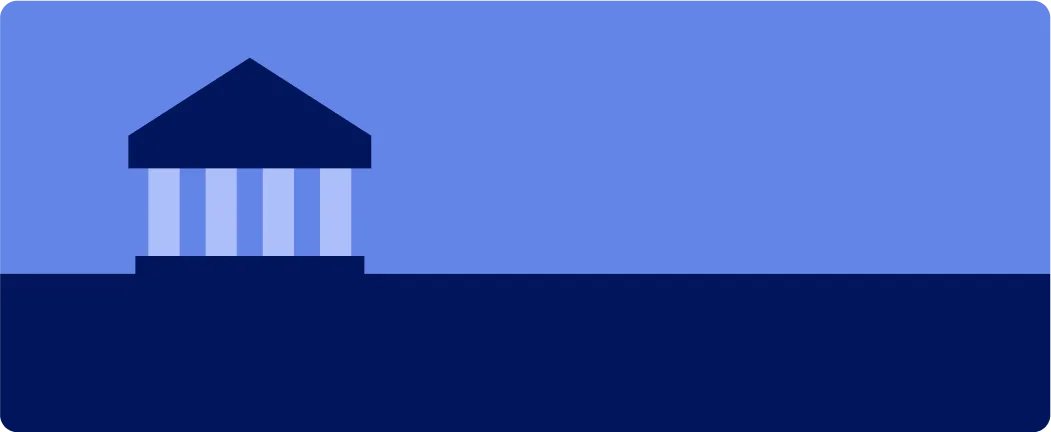

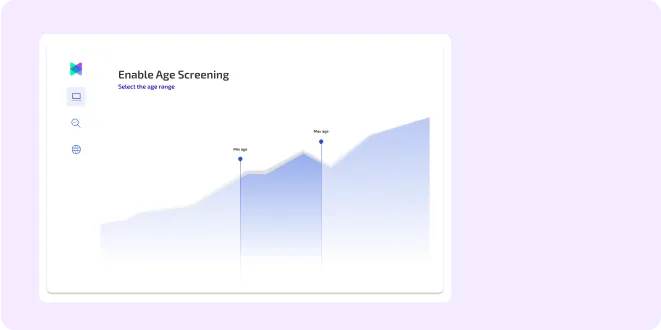
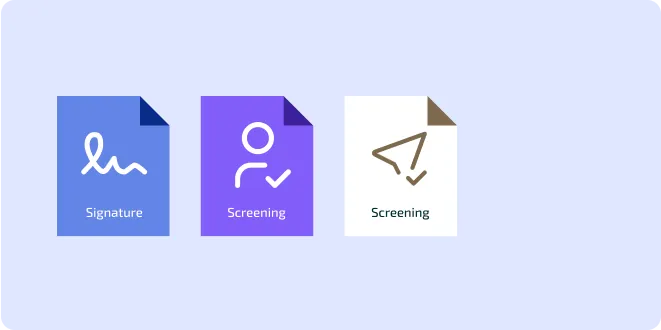
Comments
Leave a Reply
Comment policy: We love comments and appreciate the time that readers spend to share ideas and give feedback. However, all comments are manually moderated and those deemed to be spam or solely promotional will be deleted.